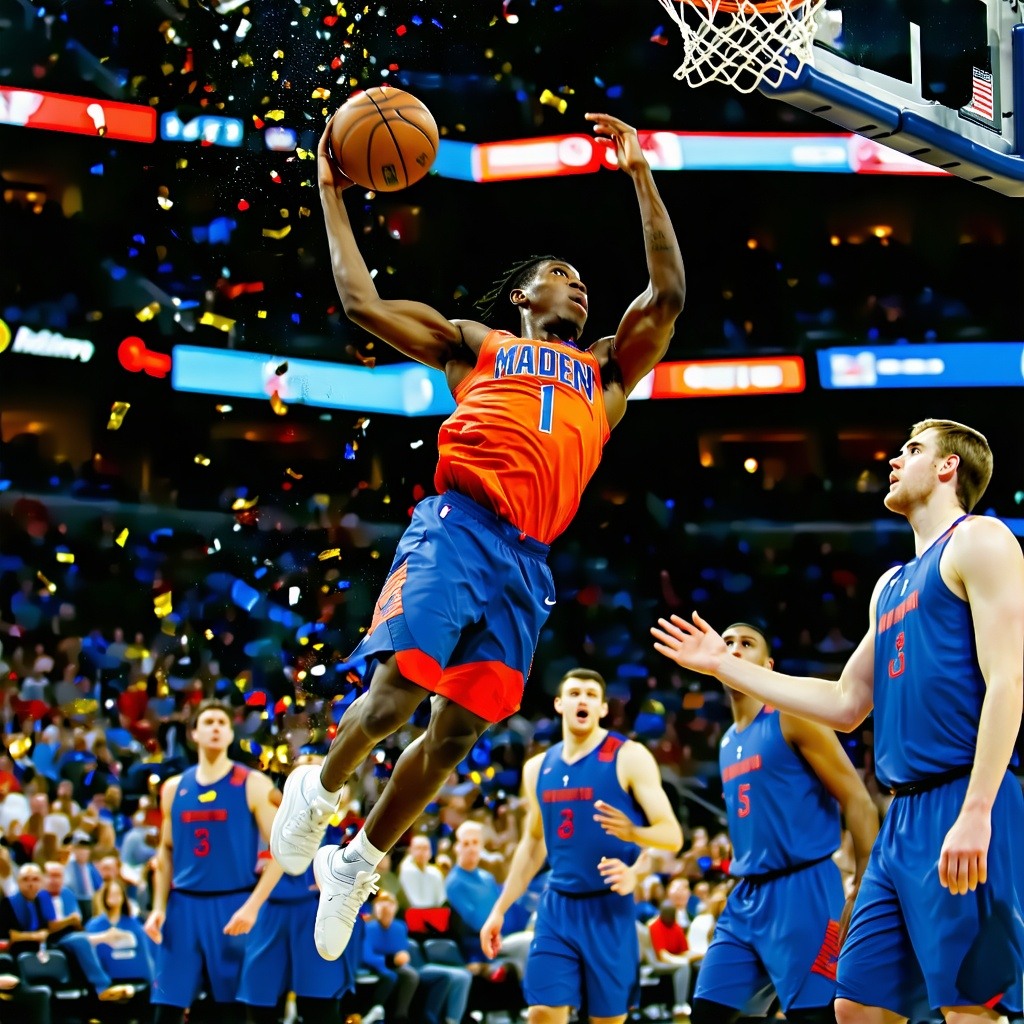
From Data Warehouses to AI Data Platforms
In today's data-driven world, organizations are constantly seeking better ways to harness their information assets. While traditional data warehouses have been the backbone of business intelligence for decades, a new paradigm is emerging: AI Data Platforms. This evolution represents not just a technological shift, but a fundamental rethinking of how we approach data integration and analysis.
The Limitations of Traditional Approaches
Traditional data warehouses rely heavily on ETL (Extract, Transform, Load) processes that often result in compromises. These systems require rigid, predefined schemas that can strip away nuanced information, reducing data fidelity. The transformation process itself is complex and resource-intensive, requiring constant maintenance as source systems evolve.
Perhaps most critically, traditional warehouses are hindered by batch processing, creating significant delays between data collection and insight generation. In today's fast-paced environment, these delays can translate to missed opportunities and slower decision-making.
The AI Data Platform Revolution
AI Data Platforms like MemberJunction take a fundamentally different approach. Rather than transforming data before storage, these platforms:
- Perform read-only replication of source data, preserving its original structure and richness
- Apply schema-on-read, allowing for greater flexibility and adaptability
- Leverage AI capabilities to interpret and analyze complex data types
- Enable real-time insights without extensive ETL processes
Moving from schema-on-write to schema-on-read fundamentally transforms how organizations think about and work with their data. Rather than forcing data into predetermined structures, AI Data Platforms adapt to the data, preserving its inherent complexity and value.
Building Intelligence on Unified Data
Beyond mere integration, AI Data Platforms serve as foundations for intelligent applications. By creating a unified data layer, organizations can develop AI-powered tools that deliver personalized experiences, automate complex workflows, and generate insights through natural language interactions.
Consider tools like Skip, which enables users to query complex datasets using conversational language. Such capabilities would be challenging to implement with traditional warehouses but become straightforward with platforms designed specifically for AI applications.
Making the Transition
For organizations considering the shift from traditional warehouses to AI Data Platforms, the benefits are compelling:
- Enhanced data fidelity through preservation of original structures
- Greater flexibility to accommodate diverse data types
- Reduced maintenance overhead by eliminating complex ETL processes
- Faster time-to-insight through real-time data access
- Lower operational costs and resource requirements
- A foundation for AI-powered applications and workflows
As data volumes continue to grow and business requirements become increasingly complex, the limitations of traditional data warehouses become more apparent. AI Data Platforms offer a path forward that aligns with the dynamic needs of modern organizations.
Want to learn more about how AI Data Platforms compare to traditional data warehouses and how they can transform your organization's approach to data?
Download our comprehensive whitepaper: "From Data Warehouses to AI Data Platforms" to explore this topic in depth by requesting here.